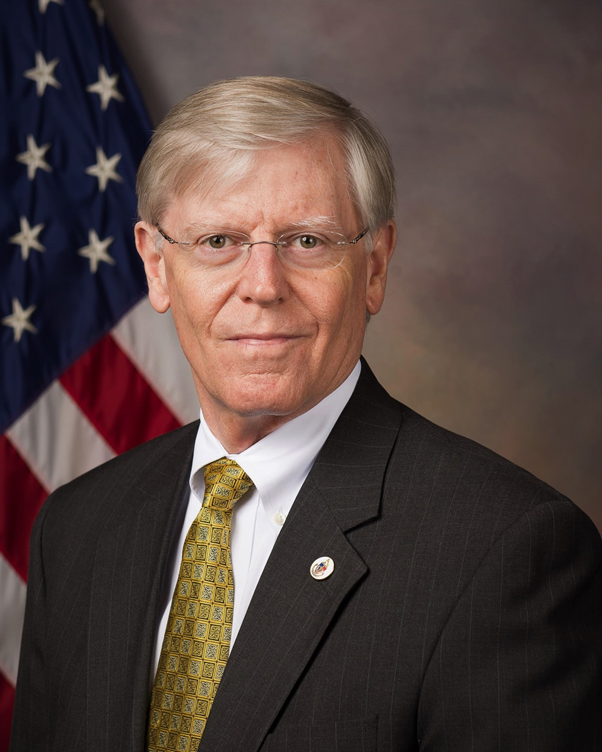
Dr. Dennis K. McBride is Director, Acquisition Innovation Research Center (AIRC), Office of the Under Secretary of Defense (A&S), Acquisition Enablers. Dennis has more than 40 years of experience at the intersection of science, technology, and public administration, including multiple levels of leadership in national security policy.
Dr. McBride served in uniform as a Naval Aerospace Experimental Psychologist at six nationally prominent high tech RDT&E laboratories and multiple headquarters – including as Program Manager at the Defense Advanced Research Projects Agency (DARPA), tri-Service lead for Operational Medicine S&T at the Naval Medical Research & Development Command, Program Officer for Modeling and Simulation, Office of Naval Research, and extensive Additional Duties (ADDU) for the Deputy Assistant Secretary of the Navy (C4I) and for the Deputy Under Secretary of Defense for Advanced Technology.
During his 20 years on active duty, Dennis was recognized for and performed several career-spanning collateral assignments, including DoD lead for Modeling & Simulation technology, and as expert in ergonomics, personnel testing, and incorporation of women into Naval Aviation. McBride retired as Navy Captain (O-6) in 1999 with 20 years of service. His decorations include among others, the Defense Superior Service Medal and the Legion of Merit – awarded for his leadership in developing and transitioning paradigm-changing technology to implementation. Three of his subsequently commercialized technologies now account for over $30B per year in revenue globally.
Throughout his Navy career, Dr. McBride taught at local universities, including Penn State, the University of West Florida, and Saint Mary’s College. Dennis was elected in 2000 by faculty as full professor, with joint appointments, in colleges of engineering and of arts & sciences at the University of Central Florida, where he taught graduate engineering and honors psych classes, and served for three years as Executive Director, Institute for Simulation and Training.
For ten years, Dr. McBride was president of the Potomac Institute for Policy Studies, providing non-partisan technical advice to Congress, the White House, and the judiciary on issues and priorities of national concern at the confluence of science, technology and public policy. He co-authored for example a decisive, amicus curiae, as invited by the U.S. Supreme Court in 2006. Dennis served a one-year term as Director (Senior Executive) of the Center for Technology and National Security Policy at the National Defense University, and three years as Assistant Vice President for Research and Co-Director of the C4I Center in the Volgenau School of Engineering at George Mason University.
From 2003 until 2013, Dr. McBride developed and taught courses after hours as adjunct professor at Georgetown University McCourt School of Public Policy (six distinct courses, including Defense, Technological Superiority & Foreign Policy, Policy Implementation, and Program Evaluation), supervised more than 50 advanced degree completions for the Army Joint Staff Internship Program, and co-created the M.S. in Science Policy and Advocacy program in public policy in the Medical School (developing and instructing six courses), graduating more than 40 Master’s students. Dr. McBride has served on multiple doctoral committees, and is a member of the Graduate Faculty at George Mason University.
Dr. McBride served for five years as Vice President for Strategy and Innovation at Source America, a $3B/year, congressionally-designated central nonprofit agency, developing jobs for people with disabilities, nationally, under the Ability One statute. He has also served as Chief Strategy Officer and Senior Scientist for NeuroRx Pharmaceutical, a for-profit corporation focused on pre- and post-market development of suicidal depression medications. Dennis also volunteers as president of the Institute for Regulatory Science, a non-profit organization in Alexandria, Virginia. He co-led and served on founding Boards for establishment of formal certifications in Modeling and Simulation (National Training Systems Association) and in Professional Ergonomics (Board of Certification in Professional Ergonomics).
In 2021 Dennis was appointed as Research Professor, Hume Center for National Security and Technology (component of the Virginia Tech National Security Institute), and as Professor of Practice, School of Policy and International Affairs at Virginia Tech, where he has collaborated across academic departments, and taught graduate course work in Homeland Security.
Dr. McBride has consulted widely for high tech R&D companies, ranging from start-ups, to legacy Defense and Intelligence R&D firms. He has been invited and continues to speak at conferences around the globe, receiving several prestigious awards such as the Australian L.P. Coombs Medal.
Professor McBride’s editorial responsibilities have included serving as Editor-in-Chief for Review of Policy Research (Policy Studies Organization/American Political Science Association), co-Editor-in-Chief for the journal, Technology, and Editorial Board (currently) for the Journal of Regulatory Science. Dennis has authored/co-authored more than 150 scientific/technical articles, and edited/authored three books.
McBride’s formal academic training includes undergraduate major in chemistry, the B.S., M.S. (quantitative/differential IO psychology), and Ph.D. (mathematical learning theory), the University of Georgia), post-doctoral MPA/M.S.P.A. in public administration, and M.S. in Systems Management (Viterbi School of Engineering, University of Southern California). Post-doctoral studies also include formal enrollment/completions at the Institute of Management, London School of Economics; graduating fourth in class, Flight Test Engineering program, University of Tennessee Space Institute/Naval Test Pilot School; Harvard Business School Program in Disruptive Strategy; and very recent completion of the Advanced Biostatistics Program at the Institute for Statistics Education in Arlington, Virginia.